The Reliability of TherAppX CORE: Today and Tomorrow
TherAppX CORE enables clinicians to navigate the ecosystem of Digital Health Tools. Here, we share a systematic approach that shows that the information shared on CORE is reliable, as reliability is necessary to make informed decisions.
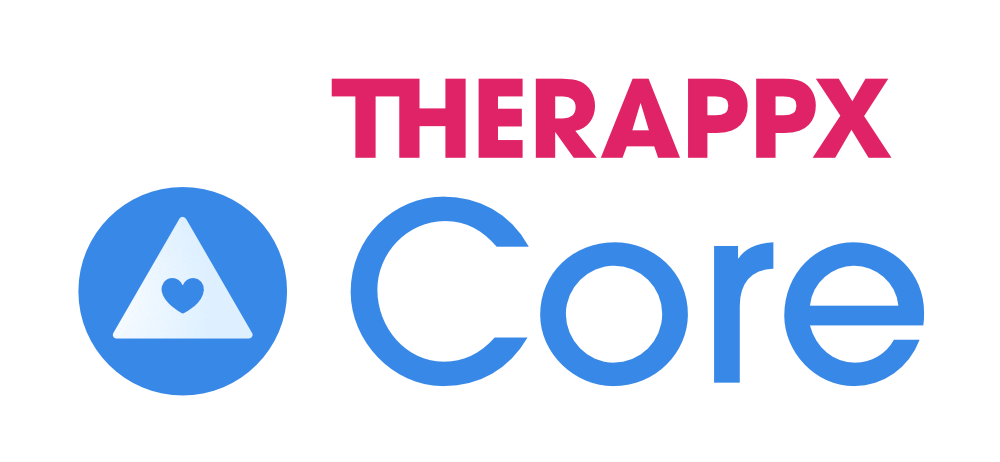
Reliability is a necessary condition for informed decisions
The need for a comprehensive platform to help clinicians navigate the impressive Digital Health Tools (DHT) ecosystem is now widely recognized, as shown by one of the latest tools developed in collaboration with the American Psychiatric Association (Lagan, Aquino, Emerson, Fortuna, Walker, & Torous, 2020). At TherAppX, we have a clear vision when it comes to assessing DHT and sharing our knowledge to the professional community. TherAppX CORE brings together over 50 key information about DHT under one roof. The review process that we have put in place provides clinicians with actionable insights to facilitate the integration of DHT into their practice. However, there are several challenges associated with generating valuable information and reliability is one of them. Researchers have already highlighted the relatively low reliability of data shared by some other platforms, such as Psyberguide, ORCHA and MindTools.io (Carlo, Ghomi, Renn, & Arean, 2019). This means that information is sometimes inconsistent between and probably within platforms. TherAppX was built from a review of existing scientific research and aims to ensure the same rigor in the technology it develops. In the following, we share some concrete actions taken by our team to ensure that the information provided by our platform is as reliable as possible, as reliability is a necessary condition for informed decisions.
How our review process works
First, a quick reminder of how our review process works (see also this article). The many components that we assess are derived from scales validated by scientific research, such as the Mobile Application Rating Scale (Stoyanov, Hides, Kavanagh, & Wilson, 2016). To this date, each DHT is reviewed by one researcher and one health professional, the two of which have received training. The researcher’s task is to assess the privacy policy, as well as other objective components related to data management, functionalities and empirical studies performed on the tool. The health professional’s task is to provide a review of the clinical potential of the DHT, using both objective and subjective judgments. Our experience with clinicians has informed us of the particular value of a number of specific information that we share on the platform, especially information around behavior change potential and clinical objectives!
Among all the information collected by health professionals, they must evaluate selected criteria from the App Behavior Change Scale or ABACUS (McKay, Slykerman, & Dunn, 2019). The ABACUS was developed from a review of more than 50 research papers on technological components promoting behavior change and new habits. With TherAppX CORE, clinicians can therefore identify the potential of a DHT to help a patient adopt a new behavior. In addition, health professionals reviewing a DHT must identify its clinical objectives, which cover the entire care continuum, from fitness and wellness, to treatment, monitoring, diagnostic and prevention (Cohen, Dorsey, Mathews, Bates, & Safavi, 2020). Given the importance of this information in making a decision on a DHT, it just seems natural to make sure that it is reliable. The systematic method that we chose goes as follows.
Assessing reliability with a systematic approach
We recruited three new health professionals undergoing studies in pharmacy, whose names will be shortened to A, B and C. During their training phase at TherAppX, A, B and C accumulated several hours of activity, consisting in a magistral introduction to the state-of-the-art in DHT assessment, along with a comprehensive overview of our evaluative framework and a carefully designed manual to help them make decisions during their reviews. In addition, they received feedback on their review of five DHT, all of which were previously reviewed by a health professional that went through the same training as A, B and C. At the end of this exhaustive training phase, we assumed their way of thinking aligned with our review framework. Next, A, B and C each reviewed twelve DHT that had never been submitted to a health professional before. We examined reliability using these reviews.
We used Cohen's Kappa statistic (k), which is a measure of inter-rater reliability and produces an estimate that takes into account agreements and disagreements that would be expected by chance (Hallgren, 2012). As a guideline, scores from 0.61 to 0.80 indicate substantial agreement, whereas scores from 0.81 to 1.0 indicate nearly perfect agreement. For each pair of health professionals, we computed an overall k for the ABACUS scale and the care objectives identified. For the A-B pair, k was 0.92 and 0.90 for the ABACUS and care continuum, respectively, while it ranged from 0.75 to 0.77 for the A-C and B-C pairs. The average k for the ABACUS (k = 0.80) and the care continuum (k = 0.79) were high, which compares favorably with what is obtained elsewhere (Lagan, Aquino, Emerson, Fortuna, Walker, & Torous, 2020). This shows that our review process leads to reliable judgments across raters and that the information displayed in TherAppX CORE can be trusted.
TherAppX CORE today and tomorrow
What can we learn from this? Some important insights can be obtained by looking at specific inter-pair reliability. Two out of three professionals had a near-perfect agreement, while the other resulted in a substantial, but slightly lower agreement. As the use of our platform has a direct impact on how clinicians interact with patients and use DHT, we have implemented measures to ensure that we keep on sharing the most reliable information. First, health professionals receive frequent feedback and we recently launched TherAppX COMMUNITY, a social platform that is aimed at clinicians and reviewers alike. The objective of this community is twofold. It will empower clinicians to integrate DHT into their practice by having access to experts, but more importantly, it will allow us to deliver additional training to our reviewers, as well as clinicians using our technology. Second, we are currently working on adding even more quality checks, including random verifications of reviews before they are shared on TherAppX CORE. We believe that reviewing DHT must be treated as an ongoing process. As such, we are currently improving the iterative nature of our review process.
DHT updates are one of the biggest challenges that regulatory instances must face (Moshi, Tooher, & Merlin, 2018). The difficulty of keeping up with software updates is also one of the most important criticisms addressed to existing platforms. The time gap between reviews and updates is a significant risk to the validity of DHT assessments. In fact, the average age of a review among Mindtools.io, Psyberguide and ORCHA is 475 days, with ORCHA being the lowest at 109 days. At TherAppX, we’ve put in place ways to track updates as they come and to distinguish between minor and major ones. In the coming months, we intend to share a timeliness metric that we are confident will be lower than other platforms.
Moshi, M. R., Tooher, R., & Merlin, T. (2018). Suitability of current evaluation frameworks for use in the health technology assessment of mobile medical applications: a systematic review. International Journal of Technology Assessment in Health Care, 34(5), 464-475. doi: 10.1017/S026646231800051X
Stoyanov, S. R., Hides, L., Kavanagh, D. J., & Wilson, H. (2016). Development and validation of the user version of the Mobile Application Rating Scale (uMARS). JMIR mHealth and uHealth, 4(2), e72. doi: 10.2196/mhealth.5849